Data science has become a cornerstone of technological advancement, playing a vital role in driving innovation and strategic decisions across numerous sectors. Its significance is especially pronounced in leading tech companies collectively known as FAANG (Facebook, Amazon, Apple, Netflix, and Google), where data scientists are essential for enhancing user experiences, optimizing operations, and spearheading product innovation. Securing a data science position in these renowned companies is highly sought after, reflecting both prestige and professional accomplishment.
Abhishek Chaurasiya is a distinguished figure in this competitive field, with over a decade of experience at prominent firms including eBay, Amazon, and Doordash. His expertise spans marketing science, product building, and consumer analytics, making his career journey a valuable blueprint for those aspiring to enter the field of data science. Notably, Abhishek co-founded Insider Training, an ed-tech startup focusing on educating emerging data science professionals, illustrating his commitment to advancing the field through education and mentorship. His role as a judge in industry competitions further highlights his dedication to nurturing new talent in this fast-paced industry.
Our conversation with Abhishek centers around a critical topic for many aspiring data scientists: carving out a successful career path in FAANG companies. Drawing on his vast experience, Abhishek offers pragmatic strategies and insights for excelling in these prestigious roles. His advice is geared toward individuals looking to make their mark in these top-tier tech organizations.
Join us as we further explore Abhishek Chaurasiya’s remarkable career in data science!
Thank you for gracing us, Abhishek. We’d love to dive right into it. In your observations, are there specific entry points or specializations within data science that provide an edge when applying to FAANG companies? For instance, is there a preference for machine learning experts over data engineers or vice versa?
To address the second part first, as it’s more straightforward, there isn’t a distinct preference for machine learning (ML) expertise over data engineering (DE) skills or vice versa. Today’s firms seek candidates who exhibit both breadth and depth in their skill sets—those who are highly knowledgeable in their own field while also familiar with other domains. Most FAANG firms currently prefer individuals who are versed in both areas, except when filling specialized roles, which are relatively uncommon.
As for the first part, there are specific skills that can provide an edge:
An emphasis on machine learning expertise is significant. FAANG companies, known for their advanced technological applications, often prioritize candidates with solid machine learning expertise. Those involved in designing and implementing machine learning models, especially in areas such as recommendation systems and natural language processing, may find themselves at a competitive advantage. Showing proficiency in machine learning frameworks, algorithms, and keeping current with the latest developments in the field is likely to be favorably regarded.
Data engineering skills are also invaluable. While machine learning is critical, FAANG companies equally value robust data engineering skills. Efficient data processing and management are foundational in any data-driven organization. Those with expertise in constructing sturdy data pipelines, enhancing data storage, and ensuring data quality may find themselves particularly in demand, given the immense scale and complexity of the data infrastructure within FAANG companies.
Lastly, interdisciplinary skill sets are advantageous. FAANG companies almost always welcome candidates with a blend of skills spanning machine learning and data engineering. Professionals who can bridge the gap between data science and engineering, effectively communicate insights, and implement scalable solutions are in high demand. Being adept in the entire data workflow, from data collection to model deployment, illustrates versatility and can set a candidate apart in the hiring process.
From your perspective, what are the most crucial technical skills or tools that a data scientist should master to be considered for a role in FAANG companies?
I believe that many young data science enthusiasts tend to overly focus on complex programming aspects and don’t allocate sufficient time to understanding the business context. In FAANG companies, the ability to solve data and analytical problems is highly valued, and this requires a deep integration of data understanding with business acumen. Grasping the business landscape is essential to align analytical efforts with the company’s overarching goals and to communicate complex findings effectively to a diverse range of stakeholders.
In the environment of FAANG firms, where vast and dynamic datasets are the norm, proficiency in data cleaning is vital. It ensures that analyses and models are built on accurate and relevant information. This contextual grasp is crucial for problem-solving, as it aids data scientists in pinpointing the key variables and crafting models that are specifically tailored to address business challenges. My advice to young data scientists is to invest time in case studies and data cleaning, in addition to model building and feature engineering. It’s this balanced approach that truly makes a difference in the field.
Beyond the technical competencies, what soft skills or attributes do you believe are vital for a data scientist to thrive in the fast-paced environment of FAANG firms?
In my view, effective ownership is one of the key soft skills sought after in FAANG companies. At such a high level, everyone is proficient in coding and statistics. However, what distinguishes outstanding data scientists from their peers is the level of ownership they take in their work. This includes not only devising solutions to existing problems but also proactively identifying new challenges that need to be addressed.
Additionally, other essential soft skills include strong and clear communication abilities. Data scientists must often explain complex data and statistical concepts to non-technical stakeholders. This makes the ability to convey intricate ideas in a digestible manner incredibly valuable. Collaboration with multiple teams is also a regular part of the job, requiring effective teamwork skills. As I mentioned earlier, possessing business acumen is crucial too. Understanding the broader business context and how it intersects with data analysis is fundamental for a data scientist’s role in these organizations.
Through “Insider Training,” how have you tailored the mock interviewing process to simulate the intense interviewing rounds of FAANG companies? What unique challenges do candidates typically face?
Good question. We strive to offer our candidates an authentic FAANG experience by providing the following:
- Utilizing ex or current FAANG data scientists as our interviewers. These individuals are typically experts and Subject Matter Experts (SMEs) with extensive interviewing experience at top tech firms, including FAANG. They are well-versed in determining which questions are appropriate for different roles, how to engage in cross-questioning with candidates, and how to interpret responses effectively.
- Incorporating a series of technical assessments and coding challenges that reflect the depth and complexity of FAANG technical interviews. This includes tasks in SQL, algorithmic problem-solving, data manipulation, and machine learning. Candidates are required to demonstrate their coding proficiency and analytical thinking.
- Simulating real-world data scenarios similar to those faced at FAANG companies. This might involve analyzing extensive and varied datasets, cleaning disorganized data, and deriving significant insights. Candidates need to show their capacity to manage the scale and intricacy of data typically found in FAANG environments.
- Conducting leadership and project-based rounds akin to those at FAANG, where we delve deeply into a candidate’s past projects. We examine the fine details and nuances of these projects, aiming to observe how candidates perform under pressure, which closely resembles the FAANG firms’ approach.
How important is having a diverse portfolio of data science projects or contributions when applying for FAANG firms? Are there certain types or domains of projects that stand out?
It’s highly important when applying for FAANG firms. It showcases a range of skills, problem-solving abilities, and the practical applications of data science across various domains. Such a portfolio demonstrates adaptability and a capacity to handle different challenges, which aligns well with the multifaceted nature of roles within FAANG companies. This not only validates technical proficiency but also illustrates the candidate’s capability to address real-world business problems, thereby enhancing the overall competitiveness of the application.
When it comes to project-based rounds at FAANG firms, it’s hard to pick, but it’s usually the projects that have had a real-world impact that are really useful. Interviewers are looking to see how much impact a candidate has driven. Projects that have shaped the direction of a company’s strategy and vision, though rare, can be the make or break for a bar-raiser interview and can at times also up-level candidates. If not that, any project where it’s easy to see impact should work.
Based on your experience and insights, have you observed any evolving trends or shifts in the data science interview processes of FAANG companies in recent years?
The data science interview process at FAANG companies has recently undergone significant changes, placing greater emphasis on practical problem-solving and the real-world application of skills. This evolution includes a tendency toward more hands-on assessments, such as coding challenges and simulated project scenarios, indicating a pivot from purely theoretical knowledge toward a practical skills evaluation. There is also an elevated focus on a candidate’s ability to communicate complex technical concepts clearly and effectively. The importance of behavioral and soft skills assessments has been amplified to gauge a candidate’s capacity for collaboration, adaptability, and functioning within dynamic, cross-functional teams. In summary, there appears to be a shift toward a more comprehensive and holistic evaluation of candidates, which is in line with the diverse nature of data science roles at FAANG companies.
Given the high competitiveness of FAANG roles, what structured preparation strategy would you recommend for aspirants, especially those transitioning from non-FAANG tech firms?
For aspirants, especially those transitioning from non-FAANG tech firms, a structured preparation strategy for FAANG data science roles should focus on mastering core technical skills, including advanced machine learning, data engineering, and coding proficiency. Gaining a deep understanding of business contexts is essential, as is practicing hands-on projects that showcase practical applications and refining soft skills to ensure effective communication. There are numerous online platforms that provide valuable resources for mock interviews and help candidates stay updated with industry trends, tools, and methodologies. Lastly, adhering to a consistent and disciplined study routine, while fostering a growth mindset and adaptability, is crucial for a well-rounded preparation strategy.
Can you share a memorable or challenging interview experience (without revealing sensitive details) from a FAANG firm that you either personally faced or know of, and what was the key takeaway from that?
I think I’d have to choose my first-ever onsite interview at Amazon for this. What I appreciated about this interview was that most of the interviewers did not rely on a predetermined set of questions; instead, they formulated questions based on how I described my projects.
They began by asking for basic details about my projects, and as we dove deeper, they presented some hypothetical data related to those projects and asked how I could solve the problem better with this new data, or how my approach to the problem might change if one of my initial assumptions were incorrect. As my responses evolved, so did the interview, shifting from technical to behavioral aspects. They explored how I would persuade my team and stakeholders to adopt a new work strategy, and how I would communicate the outcomes, whether successful or unsuccessful. I really liked that experience as it closely mirrored the reality of day-to-day life in the field.
Abhishek Chaurasiya’s expert advice offers a strategic blueprint for those aiming at data science roles within FAANG companies. Highlighting the need for a fusion of technical prowess, business acumen, and soft skills, he provides aspirants with the keys to unlock their potential in these coveted positions.
As the data science field continues to evolve, Abhishek’s insights remind us that continuous learning and adaptability are as crucial as technical skills in the journey towards success in the tech world.
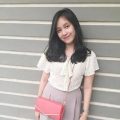
Laila Azzahra is a professional writer and blogger that loves to write about technology, business, entertainment, science, and health.