Personal data brings business value. The problem is that while businesses are trying to extract as much as possible to better their customers’ experience, government regulations -massively in sync with public sentiment- are reinforcing requirements to control how these companies use data. Under these circumstances, businesses might miss an opportunity to further improve the customer experience or may find it difficult to meet consumers’ and governments’ expectations.
But they still need it – from consumer behaviour to predictive analytics, companies consistently collect, store, and analyze massive amounts of data on their consumer base every day. Some have even built their entire business model around consumer data, whether by selling personal data to a third party or creating targeted ads. Customer data remains a top-of-mind priority for businesses who wish to remain on the floating line in a vastly competitive market.
The thing is that some data-driven organizations only now realize that defying consumers’ opinions and how their data is used may harm their brand loyalty. The latest shifts in regulations dictate a new standard where people own, manage, and benefit from the use of their PII (personally identifiable information) – allowing companies to use their data responsibly and compliantly to create positive experiences for them.
Data Anonymization: What is it?
The anonymization process ensures the removal or erasure of personally identifying information from a database. The goal is to protect the privacy of the entity, or individual the data was collected from. It involves taking sensitive personal data such as medical data or mobile metadata and removing any feature that can link it back to an individual entity.
The process of anonymization enables companies to employ large datasets for research and development reasons without compromising the privacy of their customers. Once “anonymized”, data can be:
- Processed
- Used for other purposes than that which it was collected for
- Sold
- Stored indefinitely
- Exported
In response to the latest GDPR regulations in the European Union, increasingly, more businesses are turning to data anonymization as a way to protect privacy while improving security. Being GDPR compliant not only ensures businesses will remain on good terms with the government but also help them realize the true benefits of Big Data while protecting clients and consumers alike.
Since banks, data-driven businesses, and other financial institutions are massively looking for ways to collect and process as much data as they can while remaining GDPR compliant, anonymization software from Pangeanic is improving the processing and utilization of PII (personally identifiable data) without breaking the rules.
Benefits and Applications of Anonymization
The most important benefit of using anonymization software is that the data resulting from it is not considered personal data regarding the constraints of the GDPR. Data resulting from the anonymization process isn’t identifiable anymore, which means companies will not need any permission to process it.
For example, AI-driven anonymization solutions like Pangeanic Masker can generate non-identifiable datasets that can be used and disclosed without the regulatory need for additional consent. Data is no longer considered personal information as it’s stripped of its identifying traits.
Data anonymization solutions ensure companies they:
- Comply with GDPR regulations to protect PII
- Store data more securely
- Avoid data leaks and hacks
- Share personal information safely
- Increase efficiency to save costs and effort
How does data masking work?
The main objective of data masking is generating a second version of data that cannot be easily identifiable or de-anonymized, protecting data labelled as sensitive. There are different types of data that you can protect using Pangeanic Masker, but common data types fit for data masking include:
- Personally identifiable information (PII)
- PHI: Protected health information (PHI)
- Intellectual property (ITAR)
- Payment card information (PCI-DSS)
This anonymization technique generally applies to non-production sectors like testing and software development, user training and so on – sectors that do not use actual data.
There are numerous techniques that are applied to data masking. The purpose of the user remains well-served using different techniques; however, functionalities might vary from one technique to another.
Substitution
This masking technique is similar to the shuffling technique, the only difference being that it can substitute real sets of data with fake but realistic, alternative values. For instance, client names are replaced with a random assortment of names from a list.
Shuffling
When you need to retain uniqueness when masking values, you can protect data by mixing it. Simply put, the shuffling technique aims to mix up data sets while at the same time retaining logical relationships between columns. This allows you to replace sensitive data with other values for the same attribute from a different set.
Redaction
Data redaction is a data masking practice that enables data-driven businesses to redact (mask) data by substituting or removing parts of the data sets. For instance, when sensitive data is no longer necessary, it can be replaced with generic values in the testing and development environment. That means there’s no realistic data with similar attributes to the original.
Final Thoughts
Despite the ongoing data security challenges, financial institutions and data-driven companies can and should rely on different anonymization techniques to ensure GDPR compliance, fend off sanctions, and maintain data security, a clean reputation and confidentiality.
However, most companies have neither the expertise nor the resources to deal with the production of their personal data. So, in order to prevent stiff legislative consequences, they turn to anonymization services specialized in sensitive information.
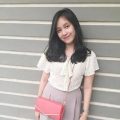
Laila Azzahra is a professional writer and blogger that loves to write about technology, business, entertainment, science, and health.